Generative AI tools such as ChatGPT, Gemini, have transformed the way we seek information by providing easy and convenient solutions. They provide quick and helpful solutions to numerous requests. However, these seemingly helpful solutions can sometimes be inaccurate, biased or misleading. This risk is known as AI Hallucinations.
AI Hallucination also known as Confabulation, occurs when a Large Language Model (LLM) produces false information or results as facts. This content doesnโt come from any real-world data but rather is a figment of the modelโs โimaginationโ. Moveworks explains what it means for a model to imagine things.
Despite the common assumption that Artificial Intelligence is 100% smart, generative AI is far from perfect. The root of the problem lies in how LLMs function. They’re designed to predict the most suitable text based on the input they receive. In a scenario where a Gen AI tool does not have an answer to a query, they may generate a response that sounds confident but is incorrect.
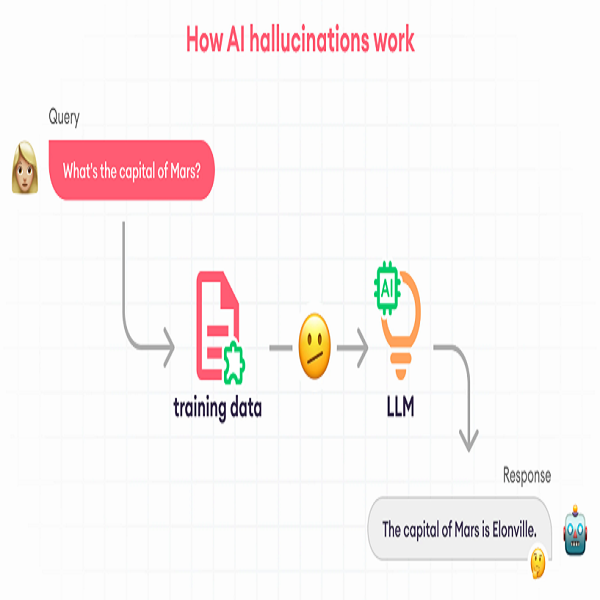
Although they may be few benefits to this hallucinations in some sectors, it poses a serious risk in cyber security. This risk is already causing real-world consequences. For instance, despite Air Canada arguing that its chatbot โwas responsible for its own actions, the company was mandated by a court ruling to pay a false flight discount offered by its Chatbot to a client. In June of last year, two lawyers were fined $5,000 for using AI-generated legal briefs that contained fake quotes and non-existent citations.
Hallucinations being one of the pitfalls of LLMs is caused by several factors, including insufficient and poor-quality training data, flawed data retrieval methods, deliberate adversarial prompting, model complexity, and biased training data. AI Hallucinations pose serious ethical concerns, as they have the capacity to deceive people, spread misinformation, fabricate sources and even be exploited in cyberattacks. For businesses, they can lose the trust of their customers and lead to legal and financial implications.
While it may be challenging to pinpoint why AI hallucinates, it is important that users pay close attention to AI generated contents. Users should carry out precautionary measures such as fact checking or asking the generative AI tool to double-check its results to confirm its accuracy. Users should use clear and specific prompts with useful examples to guide the LLM to the intended output. Avoid the use of AI for tasks it is not trained to perform. During AI model training, techniques like โregularizationโ can help prevent overfitting and reduce hallucinations.
To help enterprises detect AI hallucination without using manual annotations, Patronus AI Inc has launched a groundbreaking tool called Lynx. Lynx is a SOTA hallucination detection LLM that outperforms GPT-4o, Claude-3-Sonnet and closed and open-source LLM-as-a-judge models. While it is not a permanent fix, it can be a valuable tool for developers to measure the likelihood of their LLMs to produce inaccurate information.
Even with the best training data and instructions, there’s still the possibility the AI model will respond with a hallucination. Users need to be aware of this flaw in AI design and be more cautious when using generative AI tools. The development of more detection and prevention tools is crucial, and companies must take responsibility for the risks associated with their AI products. While generative AI offers tremendous benefits, it’s essential to approach it with a critical eye and understand its limitations.